AI
Optimizing Enterprise Asset Management with AI: Use Cases, Predictive Analytics & Best Practices
Updated 15 Apr 2025
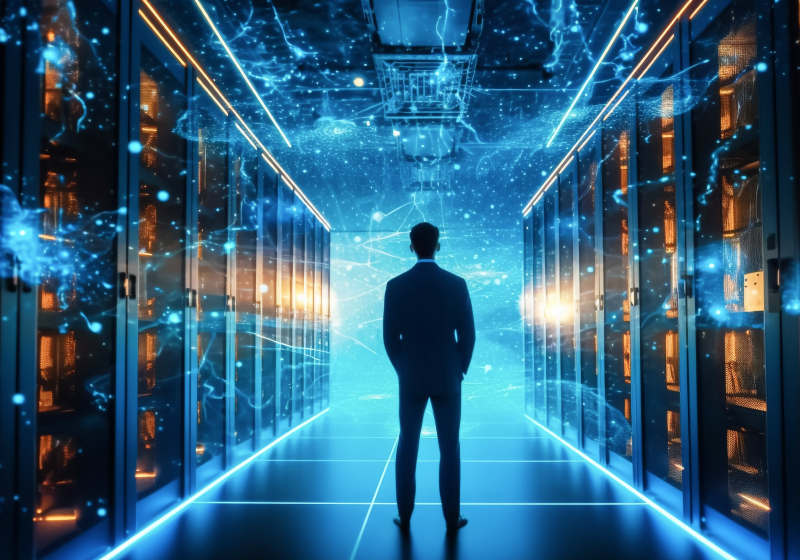
Companies across various industries now use artificial intelligence technology to enhance organizational output, minimize operational expenses and simplify management practices. AI technology has a substantial positive impact on enterprise asset management (EAM). According to the reports, the enterprise asset management market will expand to $8.4 billion through 2028, with a 10.4% annual growth rate starting from its current $5.1 billion value in 2023. The market demonstrates a quick expansion because consumers need automation tools in addition to predictive maintenance and real-time data analysis capabilities.
The implementation of AI for asset management within organizations leads to enhanced asset reliability through downtime reduction, according to Gartner findings. AI drives industrial transformation, according to the statistics presented. AI-based asset management systems advance operational effectiveness by supporting essential data-based choices needed for contemporary organizations that aim at improving sustainability and operational performance.
What is Enterprise Asset Management?
Enterprise Asset Management (EAM) defines the organized method that controls physical assets through their life cycle stages that begin during acquisition and terminate in disposal. Maintenance work and upgrade procedures, alongside compliance requirements and performance observation activities, are included in the system. The main objective of this system is the performance enhancement for assets, alongside cost reduction and life extension for all assets.
A complete EAM system merges asset information obtained from IoT sensors with maintenance log history and financial records to generate an organisation-wide asset visibility view. The operations of manufacturing and energy systems and transportation, and utilities unavoidably result in direct profitability reductions.
Transform Your Asset Management Strategy
Discover how Q3 Technologies delivers customized, scalable AI solutions tailored to your industry.
AI for Asset Management: Revolutionizing Traditional Methods
Using AI in asset management operations creates a new method of handling maintenance operations and inventory control, as well as performance optimization. Machine learning algorithms used by AI systems evaluate historical data together with real-time data for failure prediction while generating maintenance recommendations that optimize resource deployment.
The move from asset management based on response to asset management based on prediction improves stability through shorter downtimes and reduced restoration expenses, and improved asset operational performance. The data accuracy improvements, together with quicker decision-making capabilities, transform operations into agile and smarter advancement.
AI Use Cases in Asset Management
There are several AI use cases in asset management which have transformed business approaches that we will examine in this text.
1. Predictive Maintenance
AI algorithms process asset behavioral data so they can identify unusual patterns that will help predict failures before things break down. Organizations gain the ability to organize maintenance tasks in advance so they avoid unexpected equipment breakdowns.
2. Asset Performance Monitoring
AI systems, through their asset monitoring tools, operate continuously to evaluate asset performance. Businesses can launch immediate corrective measures by detecting which pieces of equipment are not performing optimally.
3. Spare Parts Optimization
AI tools optimize inventory by studying how assets are used to forecast the necessary replacement parts. The system helps business owners avoid stockpiling while also avoiding inventory depletions, which leads to cost reduction.
4. Work Order Automation
Work order creation and task distribution become automated through AI when infrastructure status and criticality levels are applied to determine workflow procedures. The system drives efficient performance from technicians while enabling them to provide prompt remedy solutions.
5. Energy Consumption Management
AI solutions monitor and evaluate energy consumption trends of equipment to locate areas with poor performance. The implementation promotes sustainability together with decreased operational costs.
6. Risk Assessment & Compliance
Historical incidents, together with regulatory data, allow AI systems to analyze asset risks through a thorough evaluation process that maintains regulatory compliance. The system reduces operational risks with its enhanced safety features.
Predictive Analytics in Asset Management
Asset management uses predictive analytics as a sophisticated capability that relies on data modelling alongside machine learning to make future asset condition predictions. Using predictive analytics allows organizations to develop from reactive strategies to predictive ones, and this increases efficiency while extending asset lifetime.
1. Real-time Data Processing
The system analyzes current sensor data to detect possible equipment breakdowns before they occur. This ensures timely maintenance interventions.
2. Failure Trend Analysis
AI technologies examine past failure trends to determine what equipment components are most vulnerable to failure. Such data-driven understanding allows organizations to create better maintenance schedules.
3. Lifecycle Cost Estimation
Predictive models help organizations calculate the full budget needs for equipment costs, along with upkeep expenditures. The system supports companies in creating optimal financial arrangements for their resources.
4. Capacity Planning
Through analytics, companies can estimate how their assets perform and how operations demand will evolve. Organizations gain better capabilities to decide if they should enhance or expand their assets.
See Why Global Clients Trust Q3 Technologies
Discover real stories of success and innovation from businesses worldwide.
Enterprise Asset Management Best Practices
Organizations achieve their highest return on investment from EAM investments when they follow enterprise asset management best practices. The following actions differentiate successful organizations from others:
1. Centralized Asset Data
An EAM system with an integrated platform unites all asset documentation within a single centralized architecture. The organization achieves better decision-making capability throughout departments by enhancing their ability to see data consistently.
2. Embrace Mobile Accessibility
Field workers receive current data and complete tasks by using mobile applications. The process increases field productivity while cutting down communication problems between teams.
3. Integrate IoT and AI
Through the unification of IoT sensors with AI-based systems, organizations can obtain precise, real-time data regarding their assets. The integrated information system allows organizations to take action on assets proactively.
4. Conduct Regular Audits
Regular audits of company assets help both the verification process and performance evaluation of physical resources. The system enables organizations to maintain proper compliance and generate correct reporting content.
5. Align with Business Goals
The company must establish asset management procedures which advance its organizational aims. When EAM initiatives match with KPIs, organizations can achieve quantifiable business results.
Experience Smart Asset Management with Q3
From predictive maintenance to real-time insights—Q3 helps you unlock the full potential of your enterprise assets.
Challenges of Poor Enterprise Asset Management
Operationally and financially, there are serious impacts that result from failing to implement correct asset management systems. The following are key effects that poor enterprise asset management has on operations:
1. Increased Downtime
Software systems that lack real-time monitoring protocols make assets fail at higher rates. The operations become disrupted, which causes productivity levels to decrease.
2. Higher Maintenance Costs
Organizations make do with reactive maintenance when they do not use predictive analytics. The result becomes inefficient operations, which require higher expenses for repairs.
3. Inaccurate Asset Records
A disordered approach to tracking assets causes both incorrect reporting together with noncompliance problems. Performance evaluation and organizational choices are negatively affected because of this situation.
4. Shortened Asset Lifespan
No proper maintenance combined with insufficient upgrades causes equipment deterioration at an accelerated rate. Organizations must spend increasingly more capital as time goes by.
Organizations must select the optimal enterprise asset management software that suits their requirements.
Choosing the Best Enterprise Asset Management Software
AI Integration
The selected software needs to include AI predictive analysis functions which enable both maintenance prognostication and equipment optimization. Such capabilities enable the addition of intelligent operational capabilities.
2. Customization Options
The software solution must adjust according to market requirements and work procedures within specific organizations. Organizational acceptance, together with return on investment, increases when users have access to customized features.
3. Real-time Reporting
The system provides comprehensive dashboards which present data analytics about asset performance, together with cost details. The system enables both strategic planning along fast operational choices.
4. Scalability
The platform has to expand with increasing organizational requirements. The platform needs to handle new asset varieties while accommodating larger information quantities.
Cost of Implementing AI in Asset Management
AI integration for EAM systems requires an up-front expenditure, but the extended advantages surpass the establishment costs. The cost of implementing AI depends on how complex the solution is, as well as which deployment model is used and what scale is deployed.
- Initial Software & Hardware Setup: Costs include purchasing AI tools, sensors, and system licenses. Cloud-based models can reduce capital expenditures.
- Customization & Integration: Adapting AI models to existing systems requires technical expertise. Integration with IoT, ERP, and maintenance tools adds to the budget.
- Staff Training: Employees need training to work with AI-driven systems. This ensures effective usage and prevents disruptions.
- Ongoing Support & Maintenance: Regular updates, system monitoring, and vendor support are part of the total cost. It ensures system reliability and performance.
Why Choose Q3 Technologies?
Q3 Technologies is a trusted leader in delivering AI-driven digital solutions for modern enterprises. Here’s why businesses choose Q3 for their enterprise asset management transformation:
- Proven Expertise in AI Solutions: Q3 Technologies brings decades of experience in deploying AI across industries. Our custom AI models are tailored for high-impact results.
- End-to-End EAM Implementation: We provide comprehensive EAM services—from consultation and development to deployment and support. This ensures seamless execution.
- Industry-specific Customization:Our solutions are built to meet the unique asset management needs of sectors like manufacturing, utilities, and logistics.
- Scalable and Cost-effective Platforms:We deliver solutions that scale with your business while keeping costs in check. Our flexible models fit both SMEs and large enterprises.
- Customer-centric Approach: At Q3, we prioritize your business goals. Our agile processes ensure faster delivery, better ROI, and continuous support.
Conclusion
Incorporating AI into enterprise asset management isn’t just a trend—it’s a necessity for organizations aiming for operational excellence, reduced costs, and competitive advantage. From predictive analytics in asset management to AI-powered decision-making, the benefits are transformative.
By choosing the best enterprise asset management software and adhering to industry best practices, organizations can overcome the challenges of poor enterprise asset management and unlock new growth opportunities.
Partner with Q3 Technologies to revolutionize your asset management strategy using AI. Experience the future of smart asset management—today.
FAQs
What is Enterprise Asset Management (EAM)?
Enterprise Asset Management refers to the structured approach of managing an organization’s physical assets throughout their lifecycle—from acquisition to disposal—while improving performance and reducing costs.
How is AI used in Enterprise Asset Management?
AI is used to analyze real-time and historical asset data, predict failures, automate maintenance tasks, and improve asset performance through data-driven decision-making.
What are the benefits of AI in asset management?
AI enhances asset reliability, reduces downtime, lowers maintenance costs, improves operational efficiency, and helps in predictive maintenance and energy optimization.
What are some common use cases of AI in asset management?
Popular AI use cases include predictive maintenance, asset performance monitoring, spare parts optimization, work order automation, energy consumption tracking, and risk assessment.
What is predictive maintenance in EAM?
Predictive maintenance uses AI algorithms to forecast equipment failures based on data patterns, allowing organizations to schedule maintenance before breakdowns occur.
How does AI support compliance in asset management?
AI analyzes regulatory and historical data to assess risks, ensure compliance, and reduce the likelihood of safety or legal violations.
What are the best practices for implementing EAM with AI?
Best practices include centralizing asset data, integrating IoT with AI, enabling mobile access, conducting regular audits, and aligning EAM initiatives with business goals.
How can I choose the best EAM software?
Look for features like AI integration, real-time reporting, customization options, and scalability. Ensure the software fits your industry-specific requirements.
What challenges do companies face with poor asset management?
Challenges include increased downtime, higher repair costs, inaccurate asset records, and reduced equipment lifespan.
Why should I choose Q3 Technologies for EAM solutions?
Q3 Technologies offers industry-specific, AI-driven EAM solutions with end-to-end implementation, scalability, and a customer-centric approach for long-term success.
Table of content
- What is Enterprise Asset Management?
- AI for Asset Management: Revolutionizing Traditional Methods
- AI Use Cases in Asset Management
- Predictive Analytics in Asset Management
- Enterprise Asset Management Best Practices
- Challenges of Poor Enterprise Asset Management
- Choosing the Best Enterprise Asset Management Software
- Cost of Implementing AI in Asset Management
- Why Choose Q3 Technologies?
- FAQs
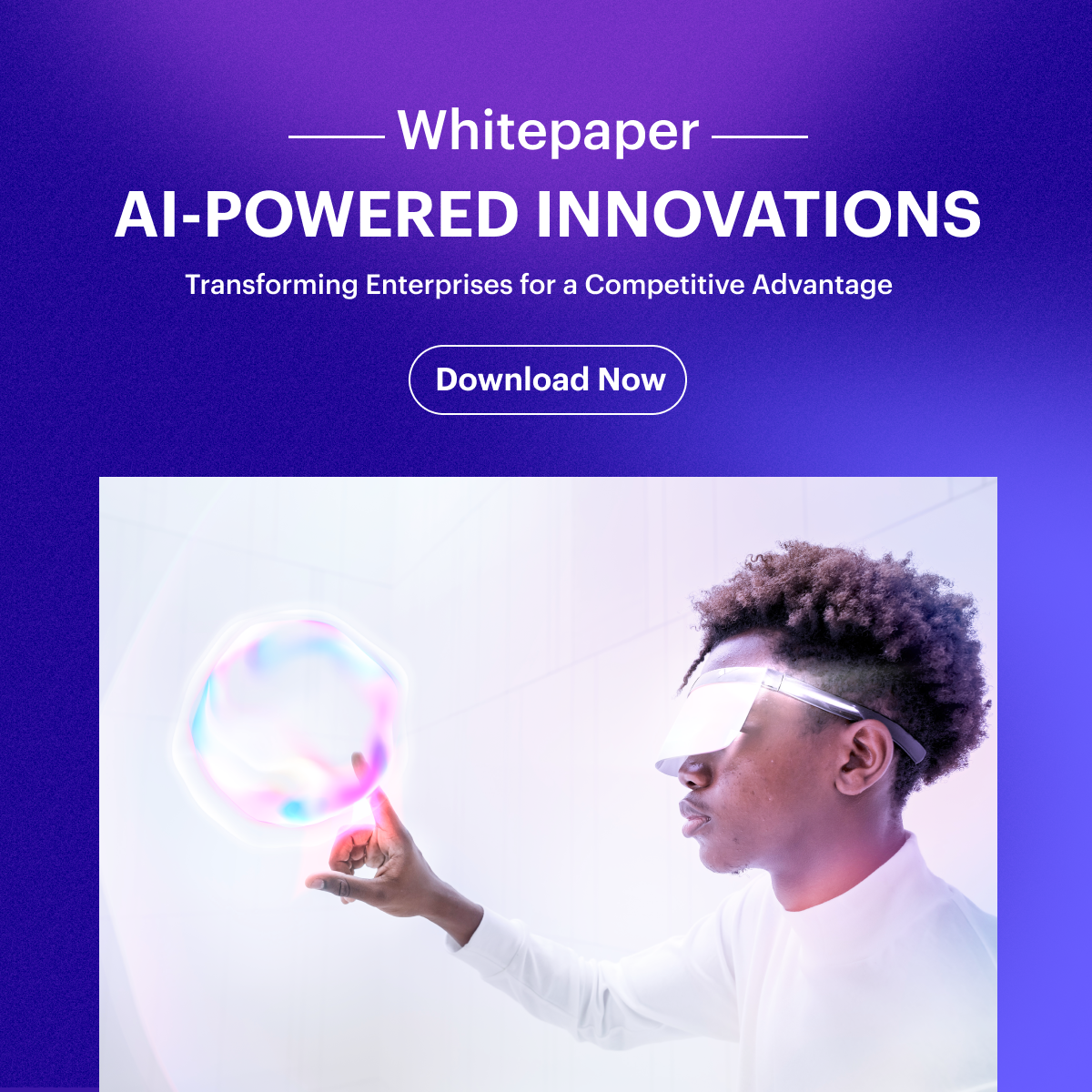